There’s no denying the technological landscape has drastically changed in the last few years. With the explosion of social media, SaaS platforms and online tools, B2B companies suddenly find themselves able to craft effective and wide-reaching strategies. The emergence of AI in B2B demand generation has only facilitated this further.
In this webinar, we’re going to look at the four main ways AI is revolutionizing how demand generation marketers are able to execute their campaigns, including:
-
- Better targeting decisions
-
- Aligning strategy with real-time content demands
-
- Delivering personalized experiences
-
- Gaining clearer insight into buyer behavior
Full transcript below.
Reading time: 21 minutes
(00:01)
Hello, my name’s Ross. I’m Head of Product at Inbox Insight. Thank you for joining us. This is our webinar: 4 Ways AI is Revolutionizing Demand Generation.
(00:12)
It’s actually part of a series, The Science of Demand Generation, which has been a few webinars in the making now, please check out inboxinsight.com for more details.
How is AI changing B2B demand generation?
(00:22)
Today we’re going to talk about, if you want predictable pipeline revenue from engaged buyers, how do you go about making a predictable formula for success from the available channels and data? The reason it’s called the Science of Demand Gen is, we want to create a test-and-learn hypothesis-based approach to success.
(00:40)
In effort of testing and experimenting with new tactics to keep pushing your strategy forward, you need to learn from the results and then confirm your findings and scale it up to affect the rest of your operation.

(00:55)
Today the theme of AI. Often we get questions from clients, as people get introduced to this topic of, how much is just hype versus how much are there real-world applications of AI. To quote Paul Daughtery, who’s chief technology and innovation Officer at Accenture:
“The playing field is poised to become a lot more competitive, and businesses that don’t deploy AI and data to help them innovate in everything they do will be at a disadvantage.”
(01:27)
Now, we surveyed 200 senior B2B marketing professionals within the Insights Professionals community. That’s the content platform that we run. And it seems that B2B marketers agree with Mr. Daugherty. We ask the question, do you perceive a threat from you new competitors in the market using AI to gain an advantage? And an overwhelming majority perceived a medium or high level threat. It’s definitely something that is impacting the day-to-day thinking of your peers in B2B marketing, and it’s definitely sort of still a relatively unknown area about how you can apply it to get the maximum results from your campaigns.
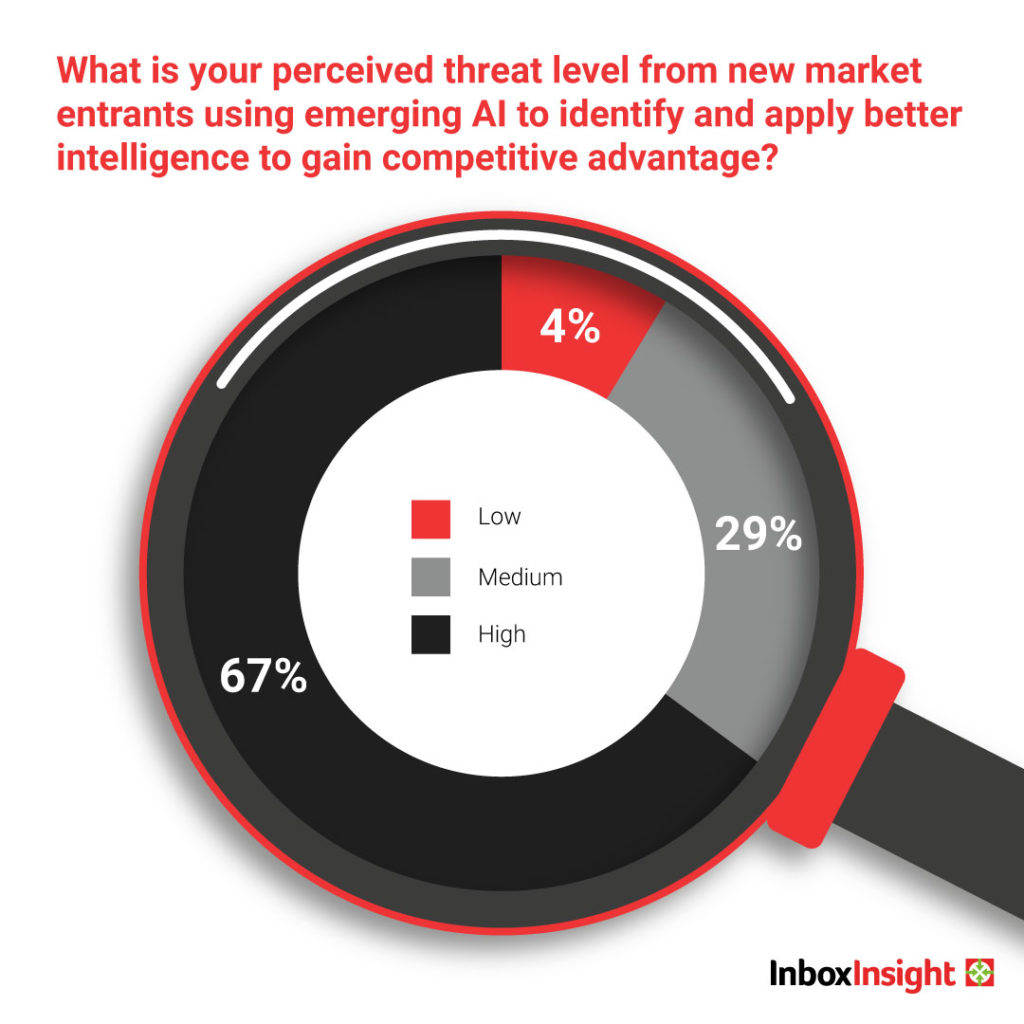
(02:07)
In an effort to talk about that, we’re going to talk about a couple of different ways that AI can be used, there are four ways that it can revolutionize your demand generation, but also some of the underlying technologies and techniques that power it. Rather than diving deep into the technology of AI, the two types that we’re going to talk about for the most part today all come back to either machine learning or natural language processing.
(02:33)
Now, machine learning is normally based around algorithms that use statistics to find patterns within data, and then use those patterns to make decisions as a person would. Over time, they get better at recognizing those patterns if given feedback, and that’s the learning part. Natural language processing is where machines begin to use the linguistic models that people do to understand either speech, like your Alexa at home, or Siri on your iPhone.
(03:06)
Or in our case, written language. The pulling down and understanding of written text. Most of these applications that we’re going to talk about today, they are using a combination of those technology types at different stages. But really, what matters is how do you apply it to your B2B business? How do you use it in a way that is going to push your marketing campaigns forward?
(03:31)
For the most part, it’s already built into various tools that are available on the market, or various partners creating customized solutions. And in that way, B2B marketing teams are quite lucky. Because it’s more accessible than you think, and already sort of lined up to some of the things that you already want to achieve in your strategy. So let’s talk about four of those.
1. Better targeting decisions
(03:52)
How can you make better segmentation and targeting decisions by using an AI-powered approach? Now, we in the research asked our panel what their biggest challenge was in trying to scale audience reach, and the overwhelming majority said it was retaining accuracy. The more they were pushing their reach out to include more B2B professionals for them to target, the harder it was to retain quality. Which makes total sense.
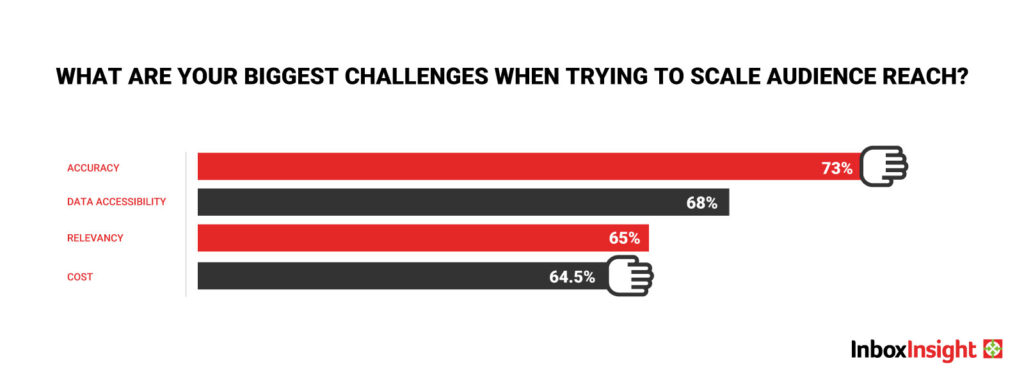
(04:21)
But in trying to solve that challenge, when we dug a little bit deeper, 71% of the audience said that they did most of their intelligence gathering in-house. And 60%, or nearly 60% of the audience, said they only use their CRM data. Over half of them identified this as a challenge for themselves. I’d say it’s probably a challenge for everybody, in that you don’t often have enough access to intelligence to really work out who the perfect, ideal customer profile is for you, and locate more of those.
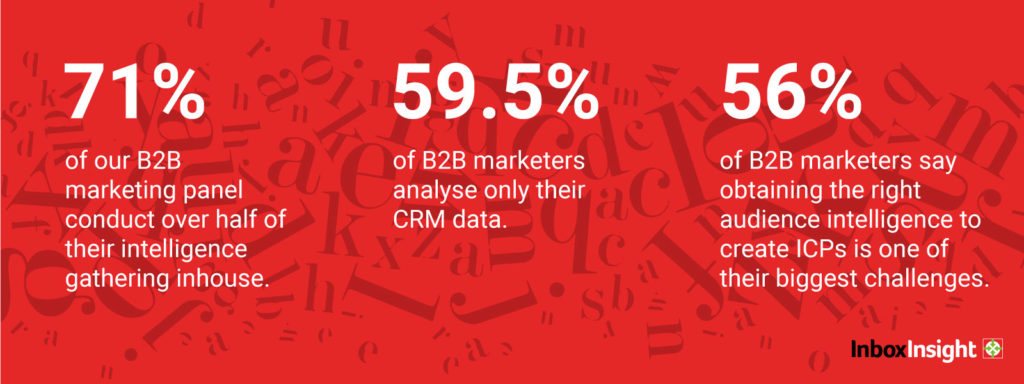
(04:54)
Before we jump into the tactics of how AI helps, let me define some of these terms. If we’re talking about total addressable market, that’s really the maximum reach for your product or category of who you would like to speak to. Who is your brand trying to target, and who could you bring into the fold? The maximum amount of those people is your total addressable market.
(05:18)
Within that, there’s an ideal customer profile. Who are you going to get the best results from, and who’s going to be most aligned to your company?
(05:27)
A competing strategy that is very popular at the moment is account-based marketing, where you’re trying to find a set of companies, like a list of companies that are already interested or fit well within the ideal customer profile. And then specifically target only those companies. And then if you manage to align your strategy around either of those, either you have a defined ICP that is already a strong product market fit and is likely to be interested, or you’ve narrowed it down to the account level and you can target those companies directly, often you limit that reach. That’s the 73% who are struggling to retain that accuracy while they scale up.
(06:07)
And that’s when we start to talk about lookalikes, or LAL, which is a lookalike list where you are beginning to take your target account list and use new techniques to find people that are similar to, in all the important characteristics, the people that you already want to target. And that’s where AI comes in.
(06:27)
Third-party lookalikes have been common and popular for a while now, in terms of cookie data and online advertising channels, like Google and Facebook will allow you to model audiences directly within their platform. When you look to first-party lookalike, so who is within your CRM data, your marketing automation data and other platforms that you have as an organization, you have to look to slightly newer technologies that rely on AI and statistical twins to work out who your ideal audience is that you already have, and how you can get more of that audience.
(07:02)
And this is where our first application of natural language processing comes in. We want to take somebody who is your best customer, those people that you have identified, and we want to use them to leap forward and identify more people like that. Normally you want to start from the company level to make sure that you are within your ideal customer profile, and then you want to dig into the decision maker level and find people who are actually interested in the topic already, or similar to people that you already have a case study or a relationship with.
(07:36)
Now let’s run through this as a workflow. This is, at a conceptual level, where you can achieve a way to make a predictable way to build out more lookalikes going forward. You begin with your customer list or a target account list that you’ve already made. I prefer starting with a customer list because it means that you’ve won business from those individuals before.
(07:58)
You use natural language processing to scan the websites of your customers. You understand in depth what it is that your customers do, or how they speak about their products and services. You can then use natural language processing to find other sites with similar keywords on the open web. You can build across lots of domains very quickly, and find people who are talking about the same things or selling the same services as your customers.
(08:24)
You can double-check this by using firmographic data, which just means company data. How large are they, what industry are they in, what technology do they have installed in their organization? That might give you some hints. And then that’s where the machine learning comes in. You combine this data together to match similar companies that have both the right content and sell the right things, and the right type of companies to get a really accurate match, while still expanding from people who are really important to your customers.
(08:53)
And then you can use, at a personal level, the information of the decision-makers within your customers, to work out the job title or persona of those people. You compare that persona to the people who are in those existing companies that you’ve just now lookaliked, and it builds out a sustainable way to expand your list and target people who are really, really relevant for you but still a much wider audience.
(09:17)
Once you begin to engage with that audience, you’ll win new customers and then that builds up your customer list and then you can repeat the profiling again.
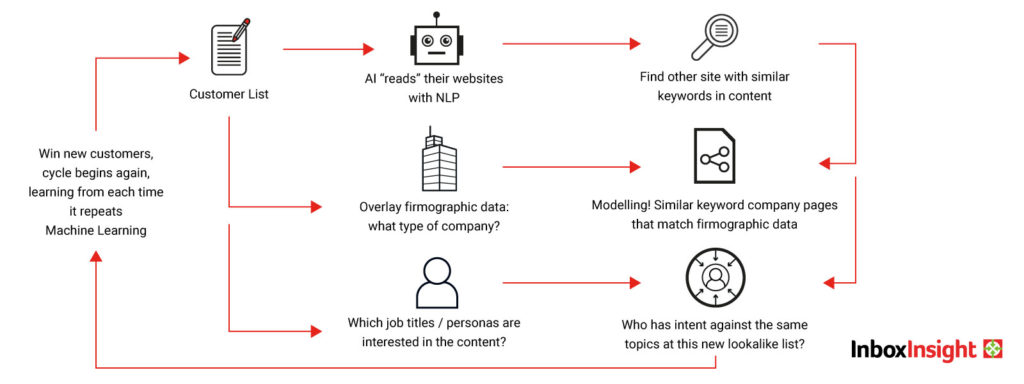
(09:29)
And let me give you a real-world example of that. I have disguised the name of the customer that we’ve worked with on this, they’re an authentication and access management vendor. And I’ve used averages just to build out a cleaner example without getting too specific. But here’s an example of how it works.
(09:45)
So the customer comes to us and says, “We have 253 companies that we want to go after on our target account list.” We would look at the domains of the people that they want to go to. We would match that across the websites and other domains that have similar keywords, and we normally average about six companies for every one company that we put in. That would take 250 to around 1500.
(10:12)
Then, in previous research we found, and Harvard Business Research, gives us this figure in particular, that there were about seven contacts in every buying decision within a B2B organization.
(10:23)
If you times your 1500 accounts by 6.8, you get nearly 10,000 new individuals that could be worth going after that are still highly relevant because they’re matched to the original target account list.
(10:36)
And then over a quarter, if you say you had an estimated 5% penetration rate into getting new prospects from that list, using your digital channels and outreach, you’d result in around 500 MQLs. So you’ve taken a list that had 250 companies, you’ve expanded that audience, and then you’ve made a sustainable way to generate pipeline off of that new audience over time, that is feeding itself.
(11:06)
The main benefits for this approach is that it does retain that relevance. It lets you build new accounts out to increase your scale while retaining that focus and that targeting, which makes you more efficient. Because you can understand the right accounts to go after, and push your sales team to follow up with people that you know are going to be more likely to buy from you.
2. Aligning strategy with real-time content demands
(11:33)
The second way that AI is sort of helping demand generation is to do with our challenge around, how do B2B marketers align their marketing strategy with real-time content demands?
(11:48)
Now, I have a rule where I like to put one over the top historical quote in every webinar. This time it’s a quote from the Art of War.
“If you know your enemy and know yourself, you need not fear the result of a hundred battles.”
(12:01)
Why am I bringing this up? The theme of this section is understanding what your competition are up to as well as what you are doing, so that you understand your content landscape. Who within your category is speaking to your customers and what are they saying to them can make a big impact in how you produce content, and how you customize content when you then go out and speak to your audience.
(12:24)
The strategic importance of that is, content intelligence means understanding the content available in the market for your customers. The competitors that are speaking to your audience may be your actual competition who are trying to sell them a different product, or it may just be the other places and sources of information that are trusted by your target audience.
(12:48)
By bringing in a mindset that uses signals to identify what people are consuming, and their preferences, you can base that on data. You can understand what people are doing and how they are engaging with content of all types within your target audience.
(13:09)
Again, we surveyed our B2B marketing panel and found that 46% of them use behavioral data in some capacity. For the most part, browsing, clicks on stuff. And 45.5% said they gained this through third-party websites as well. Which is better than just going off their own website, but it still means that over half of us are not using valuable resources to think about actually pulling down what people are doing and then understanding how they’re engaging with it to base their content strategy on hard data across their audience.
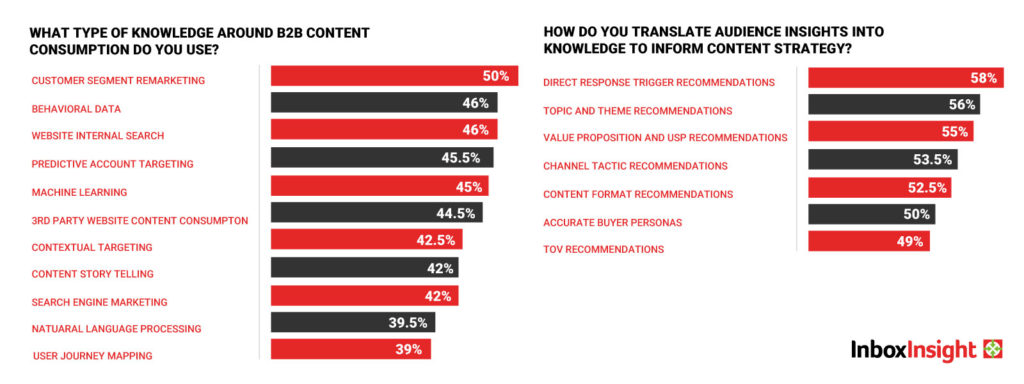
(13:41)
And in terms of how they then use that knowledge to inform their strategy, a lot of it is based around direct responses, and triggers, and remarketing. Take a single user on a content journey; what are the things that you’re presenting in front of them? Again, that’s absolutely vital, but you are missing out on predictive targeting and understanding a wider scale of how the audience as a whole is interacting with different trends and topic areas. That in turn would let you get ahead of which content you should produce in order to be saying the things that are going to attract the audience.
(14:15)
And now let’s run through how we can use AI to do this. The right application of natural language processing means that you can uncover those topic areas and those themes within a target segment. That’s about pulling down those behavioral signals, and pulling down content from sites and categorizing it so that you can understand the trends in question.
(14:36)
You would start with your audience to understand what it is that they are interested in and what topics they care about. What personas are you going to target and what content categories and keywords do you care about? And then finding other sites online which use that content, which talk about the same topic areas, including social, where you can start to begin to see how people are interacting with other brand’s content and how they’re talking about, and producing content themselves in the conversation.
(15:05)
You can gather that into one place as a series of intent data, and then you can begin to model it to find either others producing the content, or to present analytics back to you in a digestible way that says; what are people engaging with? And what content is being produced in my category?
(15:27)
Pulling this together is, for me, about trying to come up with something that gives you a real-time picture of the market and what your competition are up to, so that you can become adaptable and then you can use that to produce content that’s going to maximize engagement.
(15:47)
As an example, a couple of different content performance metrics and keywords that we track might be pulling in a target competitive set that you know is producing block content and whitepapers, guides, social content on the same themes and topics as you, and pulling down and comparing content from their sites and content from publisher sites around those same topics.
(16:10)
On the screen here, you’ve got a dashboard that we have pulled together comparing companies that provide ERP solutions and centralized cloud finance. Within that you’ve got different keywords and different performance of scores around financial planning and analysis or supply chain modernization. And you can begin to sort of rank and understand the different keywords that are popular not only within search, but the content being produced to fulfill those keywords by the competition. And then on an article by article basis, see how their content compares to yours.
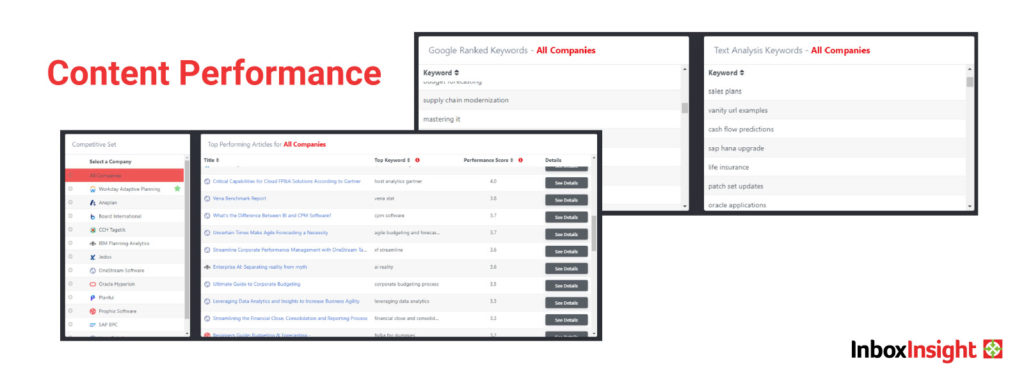
(16:47)
The reason that’s important is, you can often see with your own analytics, how your content is performing and roughly see what your strongest pieces are or your weakest pieces are. But it’s very hard to compare that to what else is out there in the market. You may think that you have a really strong, organic search performer or a really strong piece of content that always does well in your paid advertising or in your email marketing. But it might not be the article that is giving the best answer for customers, or it may have the wrong positioning, or it may have the wrong title.
(17:17)
And without giving context of the other ways that the content is being talked about by your competitors, it’s hard to be reactive with your copywriting.
(17:29)
The next example I would have is the same, but in social. So this is productivity software as a category example. And what we tend to see is trying to understand how the audience is reacting to content within social media platforms. So what level of engagement is being gathered by you and your competitors when certain hashtags and certain keywords are pulled down and organized online?
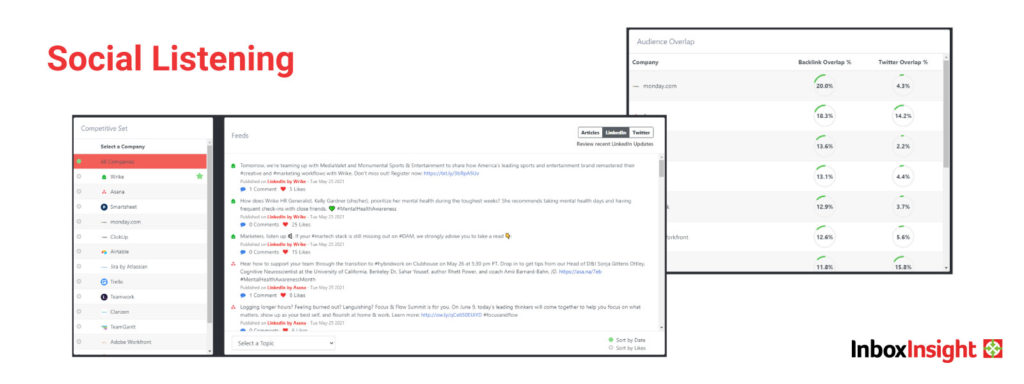
(17:52)
Again, natural language processing and keyword extraction is the AI part of this. But the content marketing strategy behind it is really trying to understand, is there a community out there that uses different terms? Is there something that the competition is saying that we should be saying or vice versa? Are we leading it? Are we generating the most engagement out there, side by side? And we should double down on that.
(18:16)
The other is trying to understand access to followers, and backlinks from certain sources, and curation of content to see the cut-through that you have to that audience and how much of an audience you share with the competition, to understand your share of voice.
(18:34)
All of this gives you vital insights into how to be adaptable with your content strategy, and how to change up what you’re saying to make sure that you are cutting through.
(18:47)
And what does this get you? The members of the panel that we asked about the three top benefits that did feel that they were getting content insight was informed content creation processes on the other side of that, at over 56%. Aligning that content that they’re producing to the buyer stages with 56% as well, and improved conversion rates at 55%. So if used correctly, content insights and content intelligence can be vital in driving the real benefits that you need from your content strategy.
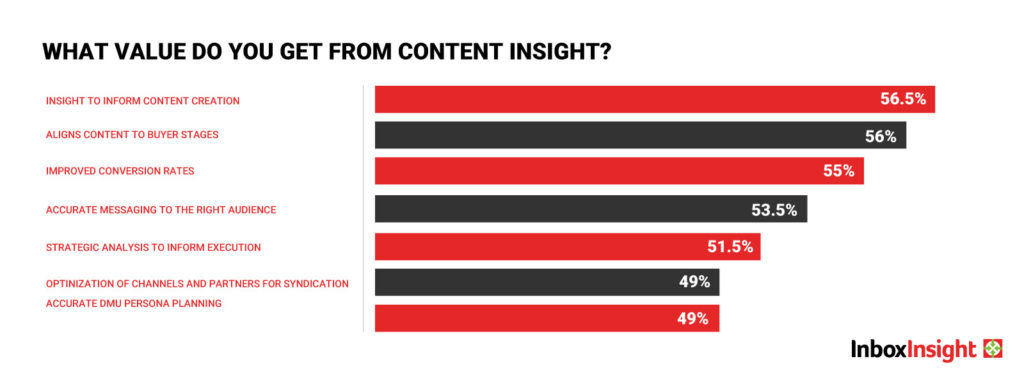
3. Delivering personalized experiences
(19:24)
With so many different potential audiences to serve, how much do you commit to creating those individual journeys and personalized experiences across all of the different audiences that you may want to target?
(19:40)
In the survey panel we found that 32.5%, which was the lowest answer, actually said that they were aiming for true one-to-one messaging. Which was originally sort of the dream of personalization, which can be really, really hard to achieve. And are more focusing on profiling and behavior triggers, which tends to come out of the box in a lot of demand generation and marketing automation platforms.
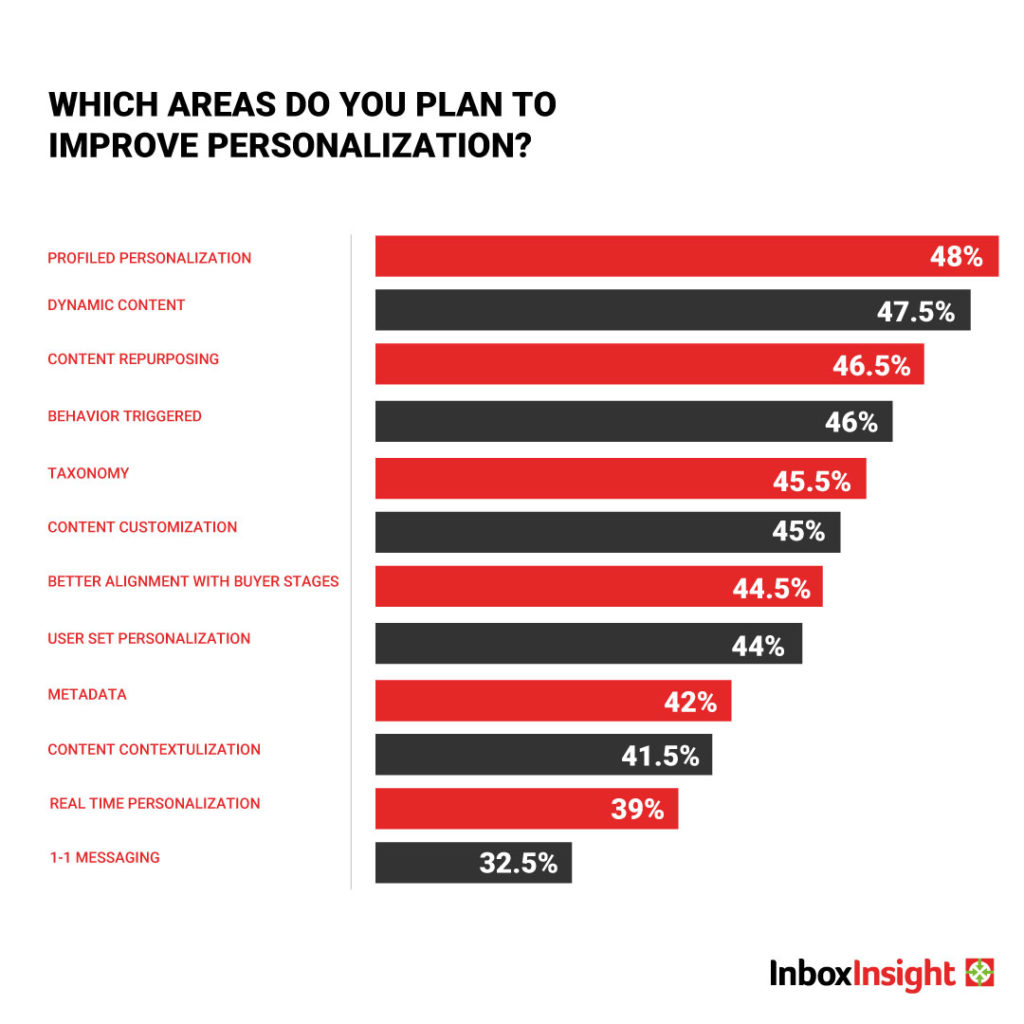
(20:05)
But can you achieve all of these different data points, aggregated together and used to commit to personalized communications? You can get pretty close, and I think that the market is going to move towards using some of the things we’ve seen in B2C and other entertainment platforms, about how you can personalize with recommendations to deliver something that is, from the content you have available, the most relevant thing to put in front of that person at the time to keep them engaged and take the next step on that content journey.
(20:41)
And the way we do this is an AI-recommended system, or recommendation engine as it’s sometimes called. Which is a tool that is used to foresee the best piece of content that should be shown to a user at any one time, and then suggest that to them. There’s two types of recommendation engines. The first is collaborative filtering, where you use click data or read-time data associated with a user, what they’re engaging with, against pieces of content. And then you use statistical analysis to find people that look like the user.
(21:17)
Finding a statistical twin, somebody that is the same in most of their features, and showing them content that the other twin has already seen. Both users like reading the same type of content. User one has read an article that user two hasn’t seen yet. I’ll show user two that article. You don’t really know what the content’s about, you are watching what the people are doing.
(21:39)
The second type is content-based filtering. That is based on tagging the content with a taxonomy, and grouping content together, sometimes by clustering, or sometimes by having people inject test data to show what content is about. When a user engages with content under a tag, they’ll be shown more, similar content under that tag in the future.
(22:03)
That could be about the topic of the content, but it could also be the type of content. Do they like watching webinars, or do they like reading whitepapers or what have you? You can build out features against the content, and then you can use those features to show the right stuff to people in the future.
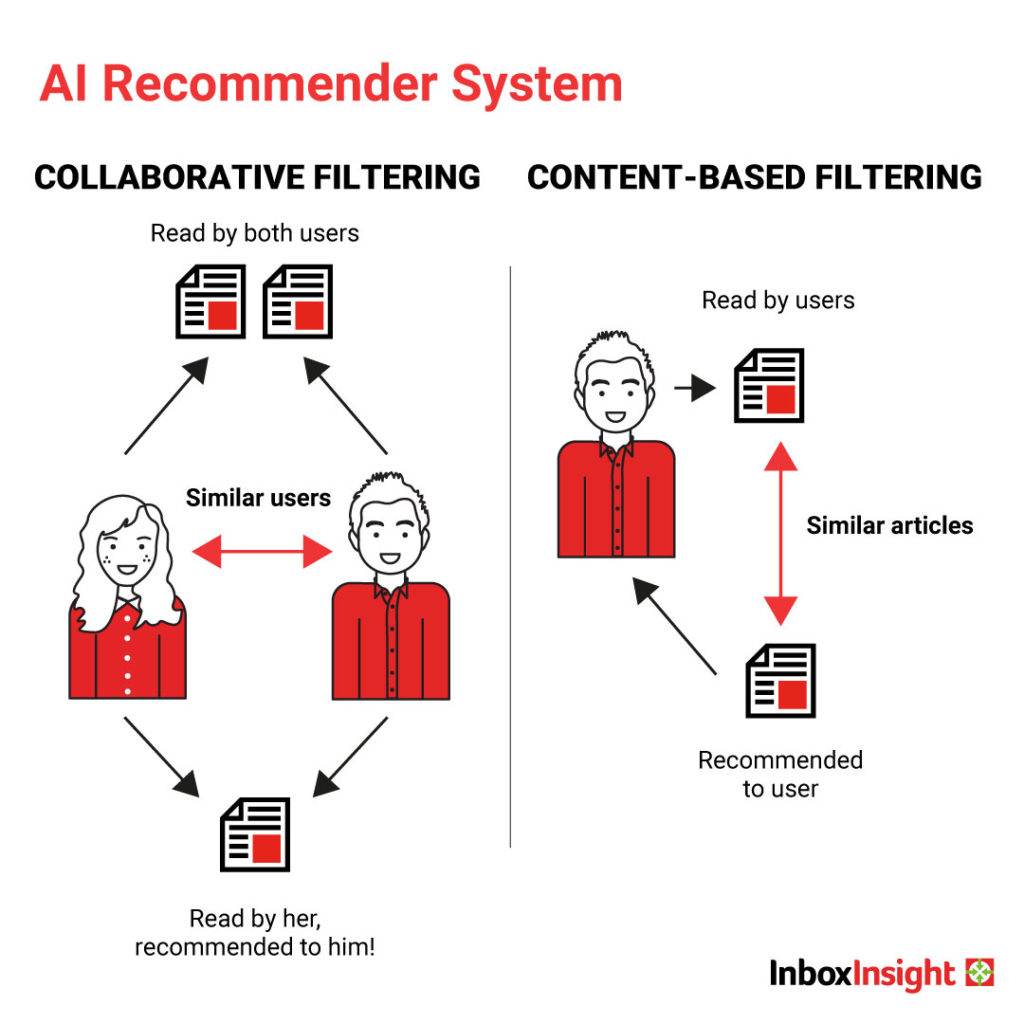
(22:18)
Most systems will have a combination of those two approaches. I’m going to do a shameless plug here, and use the example from insightsforprofessionals.com, which is our content platform that sits behind all of our campaigns, to give you an example of what that looks like from the user’s perspective.
(22:36)
Say that I am a senior business decision maker in human resources, and I am accessing content. We want to know what is the most interesting thing that you can show me at any one time? We want to pull down all of the content we have available and start to organize it based on what the user should see first.
(22:54)
Content consumption history, what have I engaged with? Or I like HR leadership, and I like cloud software, and I’m interested in managing employee performance. That content has been tagged with that with the content-filtering approach. But I also want to know what trends I want to be introduced to, what things are particularly high on the agenda within my space. What are other people who are like me interested in? And what are they clicking on, and what are they reading?
(23:22)
We want to compare across groups of people so that we can show things which are of particularly high interest from that content intelligence approach.
(23:30)
And then within first-party user data, what are the things specific to me that might really prioritize my content engagement? That might be that content needs to be aimed at a director-level audience or a project-driven audience. The content might be around my job title, around particular skills that I need, or it might be specific to an industry of the company that I work in.
(23:52)
By building up more data against an individual’s known profile, more their contact information, you can get a lot further and deeper, which is an opportunity for B2B that B2C doesn’t necessarily have to show people things that they have a particular business case need for.
(24:13)
And then once you’ve done that, once you’ve engaged your recommendation engine to understand what you should show this person next, you need to think about how you’re going to show it to them. Really, we have lots of channels available to us as B2B marketers where we can push messaging. We found that the data-driven channels that allow you to have control over the user-by-user basis, by which you show either ads or content or you engage with that person, tend to be the best for creating the queue of content and delivering it to them in a sequence. Really, you need to think about the programmatic email, paid social, and your SDRs or BDRs over the phone doing outreach.
(24:53)
How are you going to create a series of touchpoints so the best content is shown to that person, and then how to find that person in each channel? And then hopefully control yourself so that you don’t deliver too much content to that person and flood their feed. How are you going to use a sequence to deliver that to your prospect to get them to engage with content on topics that they care about, that are relevant to your brand in a repetitive way, so that they soak up and get educated by your content marketing?
(25:27)
Things to think about with this one, with content and personalization; do you have enough content? Do you have enough volume on that content underneath all of those areas? If not, you may need to think about a content atomization or repurposing strategy, which splits content apart and breaks it down into as many pieces as it can underneath an umbrella.
(25:48)
The next thing to think about is, potentially, do you have enough data to understand what the audience will care about? Do you have differentiated content experiences under each umbrella to know this is important for this type of decision-maker?
(26:05)
And then, do you have enough data to train the engine? Doing this the first time is important. But then over time, with the machine learning for the recommendation engine, you want it to get more accurate over time. You want to reinforce what it learns by putting content in front of people and watching if they engage with that content. These systems ideally get better over time. You need to think about how much data can you base this on.
(26:31)
We run thousands of campaigns across all sorts of different keywords and we often find that it can bury down into different niches, and really you need to understand and have enough content, breadth, and depth for the machine learning to learn what each user will want to see next and improve itself over time, which is that real value of AI is that it gets better and better alongside the user.
4. Gaining clearer insight into buyer behavior
(26:55)
How do B2B marketers gain clearer insight into buyer behavior? Really, they said that their top three challenges were consolidating that view of the individual, getting knowledge from the data that they have, and then actioning the insight that they get from that data. How are they actually going to use it?
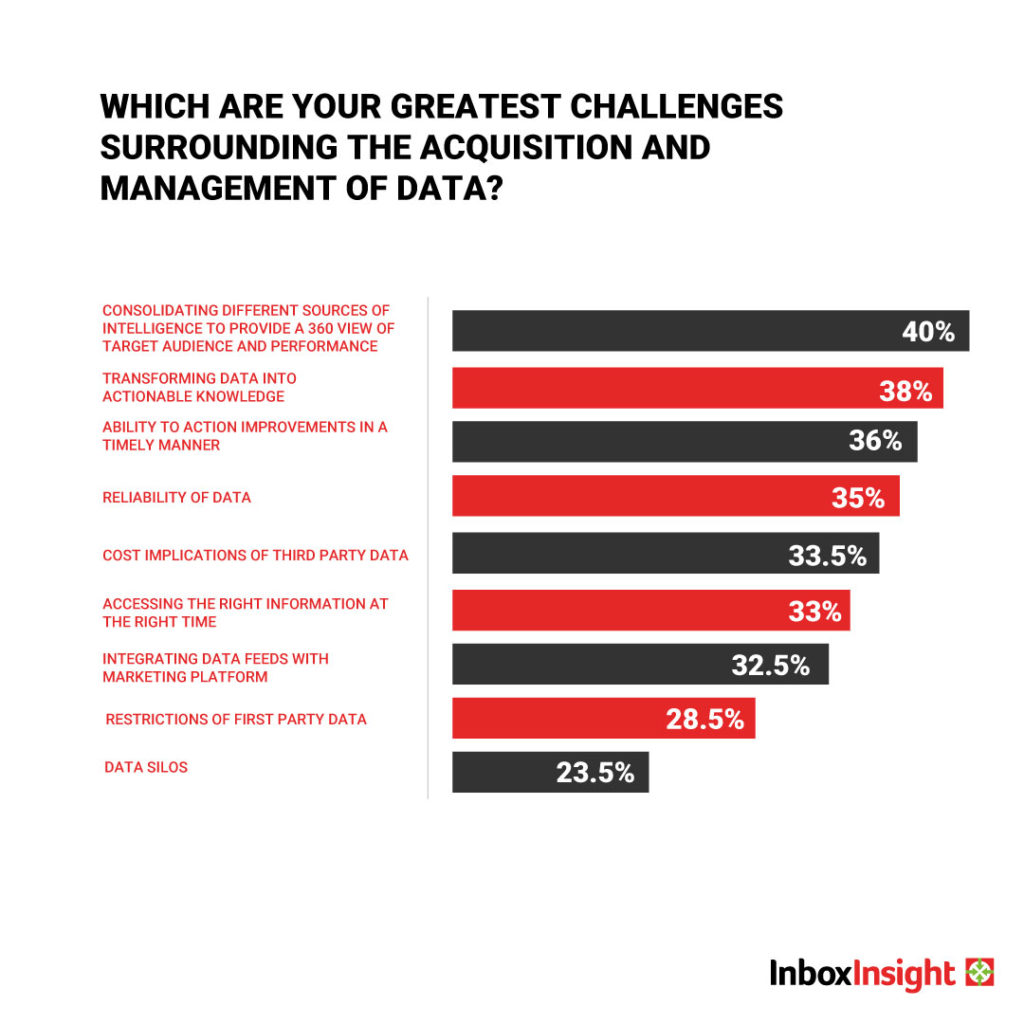
(27:17)
Here’s an example of all sorts of different touch points that you can store or engage with around a user. Really, a full 360-degree profile can be hard to achieve depending on your content stack or tech stack that you have to fulfill content to different clients and then pull down how they engage with it. It’s ideally, I think, a mindset of building out a profile against each user that is going to be useful for them as well as useful for you. And using that data to deliver them back the content is most useful for them at any time, which is going to give them a better experience with your brand.
(27:58)
One way to do that is to look at a detailed lead scoring model. Lead scoring is used often to just, in a marketing automation system, to help somebody think about how ready is this person to buy, and how much content have they engaged with? But really, it can be used as a deeper model of understanding that user and fueling all of the different systems that we’ve talked about today.
(28:20)
If you can understand and score the likelihood of relevance of the features of that person’s company, or their job title or all the information about them and score how they engage with content and what type of content they engage with, it can give you a really useful picture of who that user is and how they behave.
(28:42)
And when you have that, you can use analytics to pull that into a view which shows you a content journey. So this is an example of a content dashboard that we provide to clients as part of some of our campaigns. This example in particular shows a multinational campaign with an account base view. So we’ve dug into Capgemini, where we can see that there are multiple touchpoints over a series of weeks and months of somebody researching the journey to convert their business to the cloud.
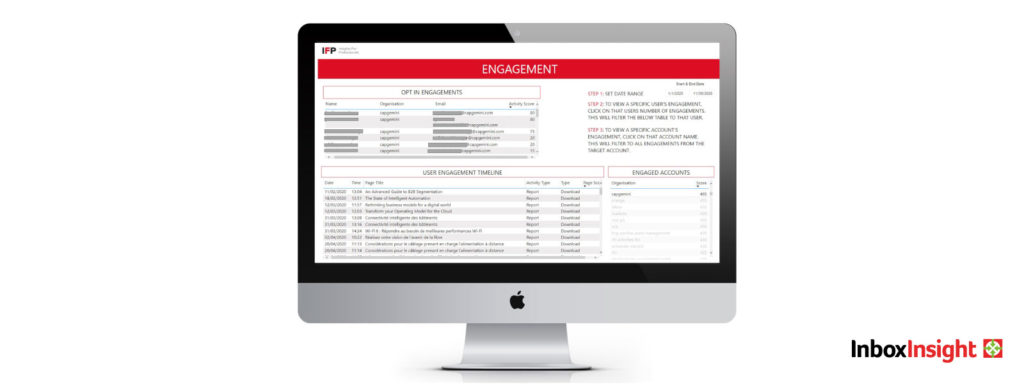
(29:13)
So if you’re seeing similar touchpoints of different topics over a period of time with multiple team members interested in that topic, it’s likely that a company has a project coming up. So it’s a good way for you to understand and deliver insight into your organization that’s actionable. For example, the sales team following up on that account first.
(29:36)
Zooming out from that view slightly, you’ve got to think about how many companies in your ecosystem or in your category at any one time are having journeys like that.
(29:45)
By using other types of AI, you can pull down more information about who’s doing that research and engaging with those content topics, and start to build a model of multiple companies doing this, which will help you target, at an individual level, the different people within that organization, so that you can personalize their customer journey and understand who has higher propensity to buy.
(30:10)
Of these 25 companies and within those companies of these five people, who is the one that sales should go after first? And that’s really the next level of how AI will revolutionize demand generation; it’s giving you that pinpoint accuracy into who you should follow up with first and how you can engage them to convert.
Thanks for your time
(30:33)
Just to recap: four ways that AI is going to revolutionize demand generation.
(30:41)
First-party lookalikes will let you scale while retaining your accuracy. Content intelligence lets you understand your competitive landscape. Hyper-personalization will help you make recommendations across multiple channels. And buyer propensity and using the insight that you’ve got will help you score accounts and convert them.
(31:00)
Thank you very much for your time. If you are interested in this type of thing, please check out inboxinsight.com where we’ve got all sorts of content and a great blog. Thanks very much, take care.
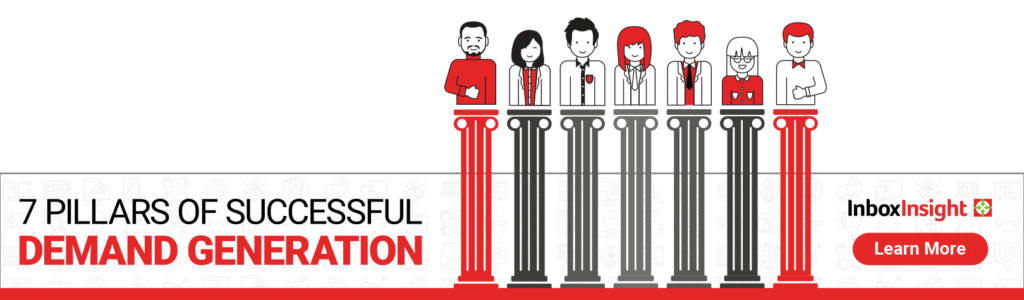